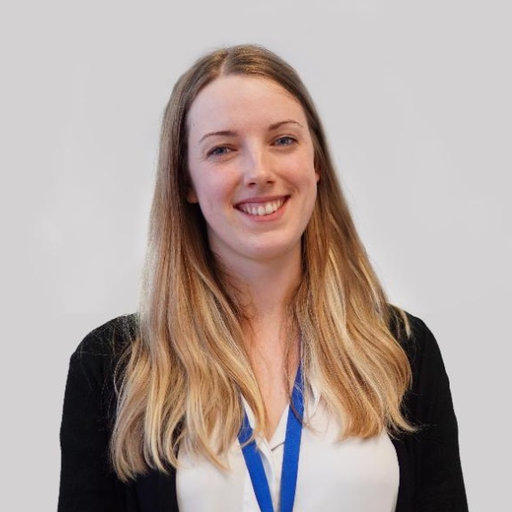
Emma Stevenson is a Marie Skłodowska-Curie Early Stage Researcher in the EU H2020 Stardust-R research network (http://www.stardust-network.eu/), based in the AIDA group in the school of Computer Systems Engineering at Universidad Politécnica de Madrid (UPM), Spain. She is researching how advanced machine learning and artificial intelligence techniques can be applied to the space sector, to make our use of space sustainable, and is also a Ph.D. student in the school of Aeronautics and Space Engineering at UPM. Her research into space sustainability is supported by secondments at the French Space Agency (CNES) in Toulouse, France, and the University of Strathclyde in Glasgow, UK, and follows from a two-year position as a Young Graduate Trainee with the European Space Agency’s Space Debris Office in Darmstadt, Germany.
Stevenson, Emma; Rodríguez-Fernández, Víctor; Urrutxua, Hodei; Camacho, David
Benchmarking deep learning approaches for all-vs-all conjunction screening Journal Article
In: Advances in Space Research, 2023.
@article{nokey,
title = {Benchmarking deep learning approaches for all-vs-all conjunction screening},
author = {Emma Stevenson and Víctor Rodríguez-Fernández and Hodei Urrutxua and David Camacho},
doi = {https://doi.org/10.1016/j.asr.2023.01.036},
year = {2023},
date = {2023-01-23},
urldate = {2023-01-23},
journal = {Advances in Space Research},
abstract = {The all-vs-all problem, for which conjunctions are screened for over all possible sets of catalogued objects, is crucial for space traffic management and space situational awareness, but is a computational challenge owing to the vast and growing number of possible conjunction pairs. In this work, we present the application of deep learning techniques to this problem, framing conjunction screening as a machine learning classification task. We investigate the performance of different input data representations and model architectures on a realistic all-vs-all dataset, generated using the CNES BAS3E space surveillance simulation framework, and consisting of 170 million object pairs over a 7-day screening period. These approaches are benchmarked against operationally used classical filters in both screening capability and computational efficiency, and the ability of deep learning algorithms to cope and aid with the scales required for current and future operational all-vs-all scenarios is demonstrated.},
keywords = {},
pubstate = {published},
tppubtype = {article}
}
Stevenson, Emma; Rodriguez-Fernandez, Victor; Minisci, Edmondo; Camacho, David
A deep learning approach to solar radio flux forecasting Journal Article
In: Acta Astronautica, vol. 193, pp. 595-606, 2022, ISSN: 0094-5765.
@article{STEVENSON2022595,
title = {A deep learning approach to solar radio flux forecasting},
author = {Emma Stevenson and Victor Rodriguez-Fernandez and Edmondo Minisci and David Camacho},
url = {https://www.sciencedirect.com/science/article/pii/S009457652100415X},
doi = {https://doi.org/10.1016/j.actaastro.2021.08.004},
issn = {0094-5765},
year = {2022},
date = {2022-01-01},
journal = {Acta Astronautica},
volume = {193},
pages = {595-606},
abstract = {The effect of atmospheric drag on spacecraft dynamics is considered one of the predominant sources of uncertainty in Low Earth Orbit. These effects are characterised in part by the atmospheric density, a quantity highly correlated to space weather. Current atmosphere models typically account for this through proxy indices such as the F10.7, but with variations in solar radio flux forecasts leading to significant orbit differences over just a few days, prediction of these quantities is a limiting factor in the accurate estimation of future drag conditions, and consequently orbital prediction. In this work, a novel deep residual architecture for univariate time series forecasting, N-BEATS, is employed for the prediction of the F10.7 solar proxy on the days-ahead timescales relevant to space operations. This untailored, pure deep learning approach has recently achieved state-of-the-art performance in time series forecasting competitions, outperforming well-established statistical, as well as statistical hybrid models, across a range of domains. The approach was found to be effective in single point forecasting up to 27-days ahead, and was additionally extended to produce forecast uncertainty estimates using deep ensembles. These forecasts were then compared to a persistence baseline and two operationally available forecasts: one statistical (provided by BGS, ESA), and one multi-flux neural network (by CLS, CNES). It was found that the N-BEATS model systematically outperformed the baseline and statistical approaches, and achieved an improved or similar performance to the multi-flux neural network approach despite only learning from a single variable.},
keywords = {},
pubstate = {published},
tppubtype = {article}
}
Stevenson, Emma; Rodríguez-Fernández, Víctor; Taillan, Christophe; Urrutxua, Hodei; Camacho, David
A deep learning-based framework for operational all-vs-all conjunction screening Proceedings Article
In: 2nd International Stardust Conference (STARCON-2), ESTEC, the Netherlands, 2022.
@inproceedings{stevenson2022_starcon2,
title = {A deep learning-based framework for operational all-vs-all conjunction screening},
author = {Emma Stevenson and Víctor Rodríguez-Fernández and Christophe Taillan and Hodei Urrutxua and David Camacho},
year = {2022},
date = {2022-11-07},
booktitle = {2nd International Stardust Conference (STARCON-2)},
address = {ESTEC, the Netherlands},
keywords = {},
pubstate = {published},
tppubtype = {inproceedings}
}
Stevenson, Emma; Rodriguez-Fernandez, Victor; Urrutxua, Hodei
Towards graph-based machine learning for conjunction assessment Proceedings Article
In: 2022 Advanced Maui Optical and Space Surveillance Technologies Conference (AMOS), Maui, Hawaii, USA, 2022.
@inproceedings{stevenson2022_amos,
title = {Towards graph-based machine learning for conjunction assessment},
author = {Emma Stevenson and Victor Rodriguez-Fernandez and Hodei Urrutxua},
year = {2022},
date = {2022-09-19},
urldate = {2022-09-19},
booktitle = {2022 Advanced Maui Optical and Space Surveillance Technologies Conference (AMOS)},
address = {Maui, Hawaii, USA},
abstract = {In the face of increasing space traffic, the deployment of large constellations, and a growing debris field, identifying potentially catastrophic collisions is an increasingly daunting and computationally challenging task. In this work, we present a novel graph-based machine learning approach for detecting conjunctions between catalogued space objects to aid in this task. Modelling conjunction events as edges between pairs of object nodes, we introduce a graphical representation of the all-vs-all scenario (so-called as it considers conjunction events between all catalogued objects, both active and debris) that is able to profit from recent advancements in Graph Neural Networks, and make a step towards efficient, machine learning based conjunction assessment. For this, we develop a methodology to predict the existence of upcoming conjunction links over a given screening period, which we frame as a graph-to-graph link prediction task, and present some initial findings that demonstrate the learning potential of the proposed approach.},
keywords = {},
pubstate = {published},
tppubtype = {inproceedings}
}
Stevenson, Emma; Martinez, Riansares; Rodriguez-Fernandez, Victor; Camacho, David
Predicting the effects of kinetic impactors on asteroid deflection using end-to-end deep learning Proceedings Article
In: 2022 IEEE Congress on Evolutionary Computation (CEC), pp. 1-8, Padua, Italy, 2022.
@inproceedings{9870215,
title = {Predicting the effects of kinetic impactors on asteroid deflection using end-to-end deep learning},
author = {Emma Stevenson and Riansares Martinez and Victor Rodriguez-Fernandez and David Camacho},
doi = {10.1109/CEC55065.2022.9870215},
year = {2022},
date = {2022-07-18},
urldate = {2022-07-18},
booktitle = {2022 IEEE Congress on Evolutionary Computation (CEC)},
pages = {1-8},
address = {Padua, Italy},
abstract = {One possible approach to deflect the trajectory of an asteroid on a collision course with the Earth, and prevent a potentially devastating impact, is the use of a kinetic impactor. The upcoming NASA DART and ESA Hera space missions will be the first to study and demonstrate this technique, by driving a spacecraft into the moon of a binary asteroid system with the aim of altering its momentum, and knocking it off course. In this work, we seek to predict critical parameters associated with such an impact, namely the momentum transfer efficiency and axial ratio of the target body, based on light curve data observed from ground before and after the impact in order to give insights into the real effect of the deflection effort. We present here our approach to this problem, which we address from a purely data-driven perspective based on simulated data provided as a part of the Andrea Milani Planetary Defence Challenge, organised by the EU H2020 Stardust-R research network in conjunction with ESA. Formulating the problem as a time series regression task, we develop an end-to-end deep learning pipeline in which we apply the latest advances in deep learning for time series, such as the use of the Transformer architecture as well as ensembling and self-supervised learning techniques. Exploiting these techniques for the challenge, we achieved second place out of the student teams, and fifth place overall without relying on any a priori knowledge of the physics of the asteroid system.},
keywords = {},
pubstate = {published},
tppubtype = {inproceedings}
}
Stevenson, Emma; Rodriguez-Fernandez, Victor; Urrutxua, Hodei; Camacho, David
Deep learning for all-vs-all conjunction detection Proceedings Article
In: 5th Workshop on Key Topics in Orbit Propagation Applied to Space Situational Awareness (KePASSA), Logroño, Spain, 2022.
@inproceedings{stevenson2022_kepassa,
title = {Deep learning for all-vs-all conjunction detection},
author = {Emma Stevenson and Victor Rodriguez-Fernandez and Hodei Urrutxua and David Camacho},
year = {2022},
date = {2022-06-01},
urldate = {2022-06-01},
booktitle = {5th Workshop on Key Topics in Orbit Propagation Applied to Space Situational Awareness (KePASSA)},
address = {Logroño, Spain},
abstract = {This paper explores the use of different deep learning techniques for detecting conjunction events in an efficient and accurate way for improved space situational awareness. Framing the problem as a machine learning classification task, we present the performance of different data representations and model architectures on a realistic all-vs-all dataset generated using the CNES BAS3E space surveillance simulation framework, and compare the approaches to operationally used classical filters in screening performance and computational efficiency. Finally, we also investigate a novel methodology for improving the performance and generalisation ability of the models using a pre-trained orbit model, ORBERT, based on self-supervised learning techniques.},
keywords = {},
pubstate = {published},
tppubtype = {inproceedings}
}
Fernandez-Mellado, Luis Sánchez; Stevenson, Emma; Rodriguez-Fernandez,; Vasile, Massimiliano; Camacho, David
An Intelligent System for Robust Decision-Making in the All-vs-All Conjunction Screening Problem Proceedings Article
In: 3rd IAA Conference on Space Situational Awareness (ICSSA), Madrid, Spain, 2022.
@inproceedings{stevenson2022_icssa,
title = {An Intelligent System for Robust Decision-Making in the All-vs-All Conjunction Screening Problem},
author = {Luis Sánchez Fernandez-Mellado and Emma Stevenson and Rodriguez-Fernandez and Massimiliano Vasile and David Camacho},
year = {2022},
date = {2022-04-01},
urldate = {2022-04-01},
booktitle = {3rd IAA Conference on Space Situational Awareness (ICSSA)},
address = {Madrid, Spain},
abstract = {The progressive increase of traffic in space demands new approaches for supporting automatic and robust operational decisions. CASSANDRA, Computational Agent for Space Situational Awareness aNd Debris Remediation Automation, is an intelligent system for Space Environment Management (SEM) intended to assist operators with the management of space traffic by providing robust decision-making support. This paper will present the automatic conjunction screening and collision avoidance manoeuvre pipeline within CASSANDRA, connecting the some of CASSANDRA's modules: Automated Conjunction Screening (ACS), Robust State Estimation (RSE), Intelligent Decision Support System (IDSS) and Collision Avoidance Manoeuvres (CAM). The pipelines allows to screen the catalogue to detect potential conjunctions, perform a detailed analysis of the encounter accounting for uncertainty (aleatory and epistemic) and new observations, provide robust decisions based on the available information and, if necessary, proposed robust optimal CAMs and analyse the impact of the new orbit on the background population. This paper will present the pipeline described above along with an example that illustrates how CASSANDRA can be used to generate robust decisions on the execution of CAMs in an automated way.},
keywords = {},
pubstate = {published},
tppubtype = {inproceedings}
}
Stevenson, Emma; Rodriguez-Fernandez, Victor; Urrutxua, Hodei; Morand, Vincent; Camacho, David
Self-supervised machine learning based approach to orbit modelling applied to space traffic management Proceedings Article
In: 11th International Association for the Advancement of Space Safety Conference (IAASS), (Virtual), Osaka, Japan, 2021.
@inproceedings{stevenson2021_iaass,
title = {Self-supervised machine learning based approach to orbit modelling applied to space traffic management},
author = {Emma Stevenson and Victor Rodriguez-Fernandez and Hodei Urrutxua and Vincent Morand and David Camacho},
year = {2021},
date = {2021-10-01},
booktitle = {11th International Association for the Advancement of Space Safety Conference (IAASS)},
address = {(Virtual), Osaka, Japan},
abstract = {This paper presents a novel methodology for improving the performance of machine learning based space traffic management tasks through the use of a pre-trained orbit model. Taking inspiration from BERT-like self-supervised language models in the field of natural language processing, we introduce ORBERT, and demonstrate the ability of such a model to leverage large quantities of readily available orbit data to learn meaningful representations that can be used to aid in downstream tasks. As a proof of concept of this approach we consider the task of all vs. all conjunction screening, phrased here as a machine learning time series classification task. We show that leveraging unlabelled orbit data leads to improved performance, and that the proposed approach can be particularly beneficial for tasks where the availability of labelled data is limited.},
keywords = {},
pubstate = {published},
tppubtype = {inproceedings}
}
Stevenson, Emma; Rodriguez-Fernandez, Victor; Urrutxua, Hodei; Morand, Vincent; Camacho, David
Artificial Intelligence for All vs. All Conjunction Screening Proceedings Article
In: 8th European Conference on Space Debris (ECSD), (Virtual), Darmstadt, Germany, 2021.
@inproceedings{stevenson2021_ecsd,
title = {Artificial Intelligence for All vs. All Conjunction Screening},
author = {Emma Stevenson and Victor Rodriguez-Fernandez and Hodei Urrutxua and Vincent Morand and David Camacho},
url = {http://oa.upm.es/67167/},
year = {2021},
date = {2021-04-01},
booktitle = {8th European Conference on Space Debris (ECSD)},
address = {(Virtual), Darmstadt, Germany},
abstract = {This paper presents a proof of concept for the application of artificial intelligence (AI) to the problem of efficient, catalogue-wide conjunction screening. Framed as a machine learning classification task, an ensemble of tabular models were trained and deployed on a realistic all vs. all dataset, generated using the CNES BAS3E space surveillance simulation framework, and consisting of 170 million object pairs over a 7-day screening period. The approach was found to outperform classical filters such as the apogee-perigee filter and the Minimum Orbital Intersection Distance (MOID) in terms of screening capability, with the number of missed detections of the approach controlled by the operator. It was also found to be computationally efficient, thus demonstrating the capability of AI algorithms to cope and aid with the scales required for current and future operational all vs. all scenarios.},
keywords = {},
pubstate = {published},
tppubtype = {inproceedings}
}
Stevenson, Emma; Rodriguez-Fernandez, Victor; Minisci, Edmondo; Camacho, David
A deep learning approach to space weather proxy forecasting for orbital prediction Proceedings Article
In: 71st International Astronautical Congress (IAC), The CyberSpace Edition, 2020.
@inproceedings{stevenson2020_iac,
title = {A deep learning approach to space weather proxy forecasting for orbital prediction},
author = {Emma Stevenson and Victor Rodriguez-Fernandez and Edmondo Minisci and David Camacho},
url = {http://oa.upm.es/64345/},
year = {2020},
date = {2020-10-01},
booktitle = {71st International Astronautical Congress (IAC)},
address = {The CyberSpace Edition},
abstract = {The effect of atmospheric drag on spacecraft dynamics is considered one of the predominant sources of uncertainty in Low Earth Orbit. These effects are characterised in part by the atmospheric density, a quantity highly correlated to space weather. Current atmosphere models typically account for this through proxy indices such as the F10.7, but with variations in solar radio flux forecasts leading to significant orbit differences over just a few days, prediction of these quantities is a limiting factor in the accurate estimation of future drag conditions, and consequently orbital prediction. This has fundamental implications both in the short term, in the day-to-day management of operational spacecraft, and in the mid-to-long term, in determining satellite orbital lifetime. In this work, a novel deep residual architecture for univariate time series forecasting, N-BEATS, is employed for the prediction of the F10.7 solar proxy on the days-ahead timescales relevant to space operations. This untailored, pure deep learning approach has recently achieved state-of-the-art performance in time series forecasting competitions, outperforming well-established statistical, as well as statistical hybrid models, across a range of domains. The approach was found to be effective in single point forecasting up to 27-days ahead, and was additionally extended to produce forecast uncertainty estimates using deep ensembles. These forecasts were then compared to a persistence baseline and two operationally available forecasts: one statistical (provided by BGS, ESA), and one multi-flux neural network (by CLS, CNES). It was found that the N-BEATS model systematically outperformed the baseline and statistical approaches, and achieved an improved or similar performance to the multi-flux neural network approach despite only learning from a single variable},
keywords = {},
pubstate = {published},
tppubtype = {inproceedings}
}
Stevenson, Emma; Lemmens, Stijn; Siminski, Jan
Deriving a Unified Space Object Catalogue for the Purpose of Space Debris Monitoring and Modelling Proceedings Article
In: 1st NEO and Debris Detection Conference, Darmstadt, Germany, 2019.
@inproceedings{stevenson2019_neosst,
title = {Deriving a Unified Space Object Catalogue for the Purpose of Space Debris Monitoring and Modelling},
author = {Emma Stevenson and Stijn Lemmens and Jan Siminski},
url = {https://conference.sdo.esoc.esa.int/proceedings/list},
year = {2019},
date = {2019-01-01},
urldate = {2019-01-01},
booktitle = {1st NEO and Debris Detection Conference},
address = {Darmstadt, Germany},
keywords = {},
pubstate = {published},
tppubtype = {inproceedings}
}
Stevenson, Emma; Virgili, Benjamin Bastida; Lemmens, Stijn; Reihs, Benedikt
Comparison of Atmosphere Models for Atmospheric Predictions Proceedings Article
In: 4th International Space Debris Re-entry Workshop, Darmstadt, Germany, 2018.
@inproceedings{stevenson2018_reentry,
title = {Comparison of Atmosphere Models for Atmospheric Predictions},
author = {Emma Stevenson and Benjamin Bastida Virgili and Stijn Lemmens and Benedikt Reihs},
url = {https://conference.sdo.esoc.esa.int/proceedings/list},
year = {2018},
date = {2018-02-01},
urldate = {2018-02-01},
booktitle = {4th International Space Debris Re-entry Workshop},
address = {Darmstadt, Germany},
keywords = {},
pubstate = {published},
tppubtype = {inproceedings}
}
Virgili, Benjamin Bastida; Lemmens, Stijn; Stevenson, Emma; Reihs, Benedikt
Statistical comparison of ISO recommended thermosphere models and space weather proxy forecasting on re-entry predictions Proceedings Article
In: 68th International Astronautical Congress (IAC), Adelaide, Australia, 2017.
@inproceedings{stevenson2017_iac,
title = {Statistical comparison of ISO recommended thermosphere models and space weather proxy forecasting on re-entry predictions},
author = {Benjamin Bastida Virgili and Stijn Lemmens and Emma Stevenson and Benedikt Reihs},
year = {2017},
date = {2017-09-01},
urldate = {2017-09-01},
booktitle = {68th International Astronautical Congress (IAC)},
address = {Adelaide, Australia},
keywords = {},
pubstate = {published},
tppubtype = {inproceedings}
}
Thomas, Alexandra; Stevenson, Emma; Gittins, Fabian W. R.; Miglio, Andrea; Davies, Guy; Girardi, Léo; Campante, Tiago L.; Schofield, Mathew
Galactic Archaeology with TESS: Prospects for Testing the Star Formation History in the Solar Neighbourhood Proceedings Article
In: The European Physical Journal Conferences, Seismology of the Sun and the Distant Stars, 2016.
@inproceedings{stevenson2016_,
title = {Galactic Archaeology with TESS: Prospects for Testing the Star Formation History in the Solar Neighbourhood},
author = {Alexandra Thomas and Emma Stevenson and Fabian W. R. Gittins and Andrea Miglio and Guy Davies and Léo Girardi and Tiago L. Campante and Mathew Schofield},
url = {https://doi.org/10.1051/epjconf/201716005006},
year = {2016},
date = {2016-07-01},
urldate = {2016-07-01},
booktitle = {The European Physical Journal Conferences, Seismology of the Sun and the Distant Stars},
keywords = {},
pubstate = {published},
tppubtype = {inproceedings}
}